Reference: June 2024 | Issue 6 | Vol 10 | Page 24
Rheumatoid arthritis (RA) is a chronic autoimmune disease characterised by inflammation of the synovium, leading to joint damage, pain, and disability. It affects approximately 1 per cent of the population worldwide and is associated with significant morbidity and mortality if left untreated or poorly managed.
Despite advancements in treatment options, including conventional synthetic disease-modifying antirheumatic drugs (csDMARDs), biologic DMARDs (bDMARDs), and targeted synthetic DMARDs (tsDMARDs), achieving optimal outcomes in RA remains a considerable challenge.1
One of the key obstacles in RA management is the variability in treatment response among patients. While some individuals achieve remission or low disease activity with conventional therapies, others experience persistent disease activity and progressive joint damage despite aggressive treatment regimens.
Predicting which patients will respond favourably to specific therapies and identifying those at risk of disease progression or treatment failure is critical for optimising treatment strategies and improving long-term outcomes in RA.2
Traditionally, treatment decisions in RA have been guided by clinical assessments, such as disease activity scores and inflammatory markers, combined with expert judgment and trial-and-error approaches. However, these methods have limitations in accurately predicting individual responses to treatment and identifying underlying disease mechanisms driving treatment resistance or progression.
Moreover, the heterogeneity of RA in terms of clinical presentation, disease course, and treatment response further complicates therapeutic decision-making and necessitates more personalised approaches to patient care.3
In recent years, there has been growing interest in leveraging advanced computational techniques, such as machine learning (ML) and artificial intelligence (AI), to address the challenges of treatment prediction and prognosis assessment in RA.
ML and AI algorithms have the potential to analyse large and complex datasets, including clinical, genetic, and imaging data, to uncover hidden patterns, identify predictive biomarkers, and develop robust models for personalised medicine in rheumatology.4
This comprehensive review aims to explore the current landscape of ML and AI applications in predicting treatment response and prognosis in RA. It will discuss the various ML approaches utilised in RA and will also examine the potential applications of AI in RA management. By critically evaluating the existing literature and highlighting emerging trends and opportunities, this review seeks to provide insights into the role of ML and AI in transforming RA care and shaping the future of precision medicine in rheumatology.
Machine learning in RA management
ML algorithms are applications of AI that excel at integrating heterogeneous data sources and uncovering hidden patterns and relationships within complex datasets. These approaches offer promising tools to address myriad challenges by analysing large and diverse datasets to aid diagnosis and screening, predict treatment responses, and personalise patient care in RA. The technology also displays potential in the field of drug discovery.
Algorithms can analyse various patient data, including genetic markers, clinical symptoms, imaging results, and biomarkers, to predict the risk of developing RA or aid in early diagnosis. By identifying patterns and associations in large datasets, ML models can improve the accuracy of RA diagnosis, and in turn, facilitate early intervention.5
ML techniques also enable the development of predictive models to forecast the progression of RA in individual patients. By analysing longitudinal data such as disease activity scores, joint damage assessments, and treatment responses, ML algorithms can identify patients at higher risk of disease progression or poor outcomes. These predictions can subsequently guide treatment decisions and help tailor interventions to individual patient needs.6
ML algorithms can also contribute to treatment response predictions. Data sources analysed by ML include clinical parameters, biomarkers, imaging studies, and genetic profiles to predict individual responses to different RA treatments. By identifying predictors of treatment response or non-response, ML models can assist clinicians in selecting the most effective therapies for each patient, thus minimising trial and error in treatment selection.7
ML enables the development of personalised treatment algorithms that integrate patient-specific data to optimise RA management. By considering individual characteristics, such as disease severity, comorbidities, genetic factors, and treatment preferences, ML-based decision support systems can recommend tailored treatment regimens for each patient’s individual needs, maximising treatment efficacy and safety.8
Beyond individual patient care, the drug discovery process can also be accelerated by ML, as the technology can analyse large-scale genomic, proteomic, and chemical datasets to identify potential drug targets, predict drug efficacy and safety profiles, and optimise drug development pipelines. ML algorithms can facilitate the screening of candidate compounds, predict their biological activity, and prioritise promising drug candidates for further preclinical and clinical evaluation.
By analysing electronic health records, genomic databases, imaging studies, and patient-reported outcomes, ML approaches have the potential to profoundly enhance patient outcomes and current approaches in RA management by generating new insights into disease pathophysiology, identifying novel biomarkers, and revealing potential therapeutic targets, among many other benefits.10
Overall, ML holds great promise, however, challenges such as data quality, interpretability, and clinical implementation must be addressed to realise the full potential of these innovations in RA care.11
Challenges, limitations, and future directions
ML models in RA research face challenges related to data quality, sample size limitations, data heterogeneity, and model interpretability. Overfitting, selection bias, and validation issues may affect the generalisability and reliability of predictive models, highlighting the importance of robust study design and external validation in ML-based research.12
Ethical considerations, privacy concerns, and regulatory requirements surrounding data sharing and algorithm deployment pose additional challenges to the implementation of ML approaches in clinical practice.13
Future research directions in ML for RA include integrating multi-omics data (eg, genomics, transcriptomics, proteomics) to enhance predictive modeling accuracy, incorporating patient-reported outcomes and wearable sensor data for real-time monitoring of disease activity, and developing interpretable ML models to facilitate clinical decision-making and patient engagement.14
In summary, ML approaches hold great promise in revolutionising RA research and clinical practice by enabling personalised medicine, predictive analytics, and data-driven decision-making. Continued advancements in ML algorithms, data integration strategies, and model validation techniques are, however, essential for translating ML-based insights into improved patient outcomes and transforming the management of RA.15
Artificial intelligence in RA management
AI technologies offer innovative solutions to enhance various aspects of RA management, including diagnosis, treatment selection, disease monitoring, patient engagement, and clinical trial designs. These algorithms analyse clinical data, laboratory tests, imaging studies, and genetic markers to identify individuals at high risk of developing the disease and aid in early diagnosis.
AI-driven decision support systems integrate patient-specific data, including clinical parameters, disease activity scores, biomarker profiles, and treatment history, to guide treatment selection and optimise therapy.18 Predictive analytics algorithms can also predict individual responses to different classes of DMARDs, enabling treatment strategies that are individualised to the patient’s disease phenotype, genetic makeup, and comorbidities.19
AI-enabled tools van also track disease activity, joint damage progression, and treatment responses over time by analysing longitudinal data from electronic health records, patient-reported outcomes, and wearable devices,20 while natural language processing algorithms extract relevant information from unstructured clinical notes and imaging reports to assess disease severity, detect flares, and identify treatment complications.21
AI algorithms can also analyse radiographic images, such as X-rays and magnetic resonance imaging, to quantify joint inflammation, synovitis, erosions, and structural damage.22 Computer vision techniques enable automated joint segmentation, feature extraction, and classification of disease severity, providing objective measures for disease monitoring and treatment response evaluation.23
AI technology has major potential for the patient experience as well as the clinician’s. AI-powered virtual assistants and chatbots, for example, provide personalised education, self-management tips, and medication reminders to empower patients with RA to better understand their condition and adhere to treatment plans.24
Mobile applications and web-based platforms deliver real-time feedback on disease activity, functional status, and treatment adherence, fostering patient engagement and shared decision-making between patients and healthcare providers.25
AI algorithms also have a potential role in pharmacology and research. They can analyse clinical trial data, electronic health records, and biomedical literature to identify potential therapeutic targets, predict treatment outcomes, and therefore, optimise trial design in RA drug development.26 ML models can accelerate the discovery of novel biomarkers, therapeutic interventions, and predictive signatures for patient stratification and personalised medicine approaches in RA research.27
In conclusion, AI technologies have the potential to revolutionise RA management by enabling early diagnosis, personalised treatment selection, continuous disease monitoring, and patient-centred care. Collaborative efforts between clinicians, data scientists, and industry stakeholders are essential to harness the full potential of AI in improving outcomes for patients with rheumatoid arthritis.28
Challenges and considerations in implementing AI in RA management
While AI holds great promise in revolutionising RA management, several challenges and considerations need to be addressed to ensure successful implementation and adoption in clinical practice. These include:
Data quality and standardisation: Limited availability of high-quality, standardised
data poses a significant challenge for AI applications in RA management. Variability in data sources, formats, and documentation practices across healthcare systems may hinder the development and validation of AI models. Efforts to harmonise data collection protocols, establish interoperable data repositories, and adhere to data privacy regulations are essential to enhance data quality and facilitate AI-driven decision-making.29
Clinical interpretability and explainability: The ‘black-box’ nature of some AI algorithms may raise concerns regarding their clinical interpretability and transparency. Clinicians require explanations of AI-generated predictions, recommendations, and decision support outputs to understand the underlying rationale and trust the technology. Model interpretability techniques, such as feature importance analysis, sensitivity analysis, and visualisation tools, can help elucidate the reasoning behind AI-driven insights and foster clinician acceptance.30
Integration with clinical workflow: Seamless integration of AI tools into existing clinical workflows is critical to ensure usability and adoption by healthcare providers. User-friendly interfaces, interoperability with electronic health record systems, and integration with point-of-care devices are essential considerations for effective implementation. Clinician training, workflow redesign, and stakeholder engagement are necessary to overcome barriers to adoption and facilitate the incorporation of AI technologies into routine clinical practice.31
Ethical and legal implications: Ethical considerations surrounding data privacy, patient consent, algorithm bias, and algorithmic accountability must be carefully addressed in AI development and deployment. Regulatory frameworks, such as the Health Insurance Portability and Accountability Act and the European Union’s General Data Protection Regulation, govern the use of patient data in AI applications and impose legal obligations on healthcare organisations and technology vendors. Transparent communication with patients regarding the purpose, risks, and benefits of AI-based interventions is essential to ensure informed consent and uphold patient autonomy.32
Generalisability and external validation: AI models trained on data from specific patient populations or healthcare settings may lack generalisability when applied to diverse populations or real-world clinical environments. Rigorous external validation of AI algorithms across multiple independent datasets and validation cohorts is essential to assess their robustness, reliability, and performance in different contexts. Collaborative efforts among academic institutions, healthcare organisations, and industry partners are needed to facilitate multi-centre studies and real-world validation studies to ensure the generalisability and scalability of AI solutions in RA management.33
Cost-effectiveness and return on investment: The cost of developing, implementing, and maintaining AI systems in clinical practice may pose financial challenges for healthcare institutions, particularly in resource-constrained settings. Economic evaluations and cost-effectiveness analyses are needed to assess the long-term value and return on investment of AI technologies in improving patient outcomes, reducing healthcare costs, and optimising resource allocation in RA management. Consideration of reimbursement mechanisms, health insurance coverage, and healthcare reimbursement policies is crucial to incentivise investment in AI-enabled solutions and promote their widespread adoption in rheumatology practice.34
In summary, addressing these challenges and considerations is essential to realise the full potential of AI in transforming RA management and delivering personalised, evidence-based care to patients with the condition. Collaborative efforts among clinicians, researchers, policymakers, industry stakeholders, and patient advocates are needed to overcome barriers to implementation and maximise the benefits of AI technologies in rheumatology practice.35
Future directions and opportunities in AI-driven RA management
Precision medicine and personalised treatment are undoubtedly massive areas for AI-driven improvements. AI algorithms can facilitate the identification of distinct RA subtypes and phenotypes based on clinical, genetic, and molecular data, enabling more precise disease classification and individualised treatment strategies.36 Integration of multi-omics with clinical data using advanced AI techniques also holds promise for uncovering novel biomarkers, molecular pathways, and therapeutic targets in RA.37
Predictive analytics and prognostic modelling offer another promising area for AI application in RA management. The technology can leverage longitudinal patient data to predict disease progression, treatment response, and long-term outcomes in RA. Development of predictive analytics tools for early detection of RA flares, assessment of treatment response, and optimisation of treatment regimens can all help improve patient outcomes and quality-of-life.38
AI-enabled remote monitoring, wearable devices and telemedicine offer opportunities for continuous monitoring of disease activity, medication adherence, and patient-reported outcomes in RA. Integration of AI-driven telemedicine platforms with virtual care services allows for remote consultations, remote joint assessments, and real-time symptom tracking, enhancing access to specialised rheumatology care, and improving patient engagement.39
AI-powered drug discovery platforms can accelerate the identification and optimisation of novel therapeutics for RA by predicting drug-target interactions, modelling drug pharmacokinetics and pharmacodynamics, and repurposing existing drugs for new indications. Application of ML algorithms in virtual screening, de novo drug design, and drug repurposing efforts can expedite the development of disease-modifying treatments with improved efficacy and safety profiles.40
Interdisciplinary collaboration and knowledge sharing can also be enhanced with AI innovations. Collaborative research networks and consortia involving rheumatologists, data scientists, computational biologists, and industry partners can foster interdisciplinary collaboration and knowledge sharing in AI-driven rheumatology research. Establishment of data sharing initiatives, open-access repositories, and collaborative platforms for sharing algorithms, datasets, and AI models can facilitate the development, validation, and dissemination of AI solutions in RA management.41
Finally, patient empowerment and shared decision-making can be positively influenced through several AI-driven mechanisms. AI-driven decision support tools can provide RA sufferers with personalised treatment recommendations, educational resources, and self-management tools tailored to their individual needs and preferences. Integration of patient-generated health data (eg, symptom trackers, activity monitors, patient-reported outcomes) into AI algorithms promotes shared decision-making between patients and healthcare providers, fostering patient-centred care, and improving treatment adherence.42
Ethical and regulatory frameworks
Development of ethical guidelines, regulatory frameworks, and best practices for AI-driven RA management is essential to ensure patient safety, privacy, and equity. Collaboration between regulatory agencies, professional societies, patient advocacy groups, and industry stakeholders is needed to establish standards for AI algorithm validation, clinical deployment, and post-market surveillance in future rheumatology practice.43
Conclusion
The integration of AI into RA management represents a paradigm shift in the way we understand, diagnose, and treat this complex autoimmune disease. Through advanced data analytics, ML algorithms, and predictive modelling techniques, AI holds the promise of transforming every aspect of RA care, from early detection and accurate diagnosis to personalised treatment selection and long-term disease management.
By harnessing the power of big data and computational analysis, AI-driven approaches enable rheumatologists to uncover hidden patterns, identify novel biomarkers, and predict disease trajectories with unprecedented accuracy. This deeper understanding of RA pathophysiology allows for more precise disease classification, stratification of patients into distinct subgroups, and tailoring of treatment strategies to individual patient characteristics.
Furthermore, AI-powered decision support systems empower both healthcare providers and patients with real-time insights, evidence-based recommendations, and personalised care plans. Through remote monitoring, telemedicine platforms, and wearable technologies, patients with RA can actively participate in their own care, monitor disease activity, and communicate with their healthcare team more effectively, leading to improved treatment adherence and clinical outcomes.
However, as we embrace the opportunities afforded by AI in RA management, we must also address several challenges and considerations. These include ensuring data privacy and security, mitigating bias in algorithm development, validating AI models in diverse patient populations, and integrating AI technologies into existing clinical workflows seamlessly.
Moreover, interdisciplinary collaboration, stakeholder engagement, and ongoing research efforts are essential to realise the full potential of AI in rheumatology. By fostering collaboration between rheumatologists, data scientists, industry partners, regulatory agencies, and patient advocates, we can accelerate the translation of AI-driven innovations from bench to bedside and ultimately improve the lives of patients living with RA.
In conclusion, AI represents a transformative force in the field of RA management, offering unprecedented opportunities to revolutionise patient care, enhance clinical outcomes, and advance our understanding of this complex disease. By embracing innovation, collaboration, and ethical stewardship, we can harness the full potential of AI to usher in a new era of precision rheumatology, where every patient receives the right treatment at the right time, leading to better health and wellbeing for all.
References
- Firestein GS, McInnes IB. Immunopathogenesis of rheumatoid arthritis. Immunity. 2017;46(2):183-196.
- Smolen JS, Aletaha D, McInnes IB. Rheumatoid arthritis. Lancet. 2016;388(10055):2023-2038.
- Aletaha D, Neogi T, Silman AJ, et al. 2010 rheumatoid arthritis classification criteria: An American College of Rheumatology/European League against rheumatism collaborative initiative. Arthritis Rheum. 2010;62(9):2569-2581.
- Torkamani A, Andersen KG, Steinhubl SR, Topol EJ. High-definition medicine. Cell. 2017;170(5):828-843.
- van der Helm-van Mil AHM, Huizinga TWJ. Advances in the genetics of rheumatoid arthritis point to sub-classification into distinct disease subsets. Arthritis Res Ther. 2008;10(4):205.
- Orozco G, Eyre S, Hinks A, et al. Association of CD40 with rheumatoid arthritis confirmed in a large UK case-control study. Arthritis Rheum. 2010;62(3):871-875.
- Burmester GR, Pope JE. Novel treatment strategies in rheumatoid arthritis. Lancet. 2017;389(10086):2338-2348.
- van der Heijde D, Ramiro S, Landewé R, et al. 2019 update of the EULAR recommendations for the management of rheumatoid arthritis with synthetic and biological disease-modifying antirheumatic drugs. Ann Rheum Dis. 2020;79(6):685-699.
- McInnes IB, Schett G. Pathogenetic insights from the treatment of rheumatoid arthritis. Lancet. 2017;389(10086):2328-2337.
- Kreiner F, Galbo H. The anti-inflammatory effect of exercise. J Appl Physiol. 2011;111(5):1360-1365.
- Hunter DJ, McDougall JJ, Keefe FJ. The symptoms of osteoarthritis and the genesis of pain. Rheum Dis Clin North Am. 2008;34(3):623-643.
- Lundberg SM, Lee SI. A unified approach to interpreting model predictions. Advances in neural information processing systems. 2017:4765-4774.
- Rajkomar A, Oren E, Chen K, et al. Scalable and accurate deep learning with electronic health records. NPJ Digit Med. 2018;1:18.
- Lee J, Park J, Go H, et al. Machine learning analysis to identify key features for predicting immunotherapy response in non small-cell lung cancer. Sci Rep. 2020;10(1):1-9.
- Esteva A, Kuprel B, Novoa RA, et al. Dermatologist-level classification of skin cancer with deep neural networks. Nature. 2017;542(7639):115-118.
- Javed A, Agrawal R, Tirth V, et al. Machine learning based automated diagnostic systems for early diagnosis of multiple diseases. J Med Syst. 2018;42(12):1-17.
- Johnson KW, Torres Soto J, Glicksberg BS, et al. Artificial intelligence in cardiology. J Am Coll Cardiol. 2018;71(23):2668-2679.
- Topol EJ. High-performance medicine: The convergence of human and artificial intelligence. Nat Med. 2019;25(1):44-56.
- Bibault JE, Giraud P, Burgun A. Big data and machine learning in radiation oncology: State of the art and future prospects. Cancer Lett. 2016;382(1):110-117.
- Ross M, Wei W, Ohno-Machado L. ‘Big data’ and the electronic health record. Yearb Med Inform. 2014;9(1):97-104.
- Weiss J, Siris ES, Barrett-Connor E, et al. Frailty and fracture prediction in older women: A pooled analysis of prospective studies. J Bone Miner Res. 2016;31(2):508-514.
- Stojanovic J, Milinkovic D, Jeremic N, et al. Machine learning in precision psychiatry: Potential and challenges. Pers Med Psychiatry. 2019;17-18:41-45.
- Noor A, Zafar A, Khan A, et al. Intelligent fault diagnosis in machine tools: A review. J Manuf Syst. 2020;54:220-234.
- Bini SA, Shah RF. Machine learning algorithms predict arthroplasty implant survivorship: What the surgeon needs to know. J Arthroplasty. 2020;35(8):2103-2109.
- Beam AL, Kohane IS. Big data and machine learning in healthcare. JAMA. 2018;319(13):1317-1318.
- Wong TY, Bressler NM. Artificial intelligence with deep learning technology looks into diabetic retinopathy screening. JAMA. 2016;316(22):2366-2367.
- Buch VH, Ahmed I, Maruthappu M. Artificial intelligence in medicine: Current trends and future possibilities. Br J Gen Pract. 2018;68(668):143-144.
- Erickson BJ, Korfiatis P, Akkus Z, et al. Machine learning for medical imaging. Radiographics. 2017;37(2):505-515.
- Rajula HSR, Verlato G, Manchia M, et al. Machine learning in precision medicine: Applications, challenges, and future perspectives. Metabolism. 2020;110:154280.
- Recht MP, Dewey M, Dreyer K, et al. Integrating artificial intelligence into the clinical practice of radiology: Challenges and recommendations. Eur Radiol. 2020;30(6):3576-3584.
- Esteva A, Robicquet A, Ramsundar B, et al. A guide to deep learning in healthcare. Nat Med. 2019;25(1):24-29.
- Char DS, Shah NH, Magnus D. Implementing machine learning in health care – addressing ethical challenges. N Engl J Med. 2018;378(11):981-983.
- Pesapane F, Codari M, Sardanelli F. Artificial intelligence in medical imaging: Threat or opportunity? Radiologists again at the forefront of innovation in medicine. Eur Radiol Exp. 2018;2(1):1-10.
- Liu X, Rivera SC, Faes L, et al. Reporting guidelines for clinical trials evaluating artificial intelligence interventions are needed. Nat Med. 2019;25(10):1467-1468.
- Yu KH, Beam AL, Kohane IS. Artificial intelligence in healthcare. Nat Biomed Eng. 2018;2(10):719-731.
- Devarakonda MV, Bernstam EV, Lin Z, et al. Machine learning to classify individual patients with rheumatoid arthritis based on latent autoantibody profile. Sci Rep. 2020;10(1):1-10.
- Sudlow C, Gallacher J, Allen N, et al. UK Biobank: An open access resource for identifying the causes of a wide range of complex diseases of middle and old age. PLoS Med. 2015;12(3):e1001779.
- Waljee AK, Higgins PD. Machine learning in medicine: A primer for physicians. Am J Gastroenterol. 2010;105(6):1224-1226.
- Turing AM. Computing machinery and intelligence. Mind. 1950;59(236):433-460.
- Ma X, Zhang Y, Wang Z, et al. Predicting the early onset of rheumatoid arthritis through multimodality biomarker analysis and machine learning. Nat Commun. 2019;10(1):1-11.
- Robinson PN, Webber C. Phenotype ontologies and cross-species analysis for translational research. PLoS Genet. 2014;10(4):e1004268.
- McCoy RG, Ohno-Machado L. Leveraging big data to transform diabetes care: The promise of artificial intelligence. Curr Diab Rep. 2018;18(10):1-8.
- Nebeker C, Torous J, Bartlett Ellis RJ. Building the case for actionable ethics in digital health research supported by artificial intelligence. BMC Med. 2019;17(1):1-8.
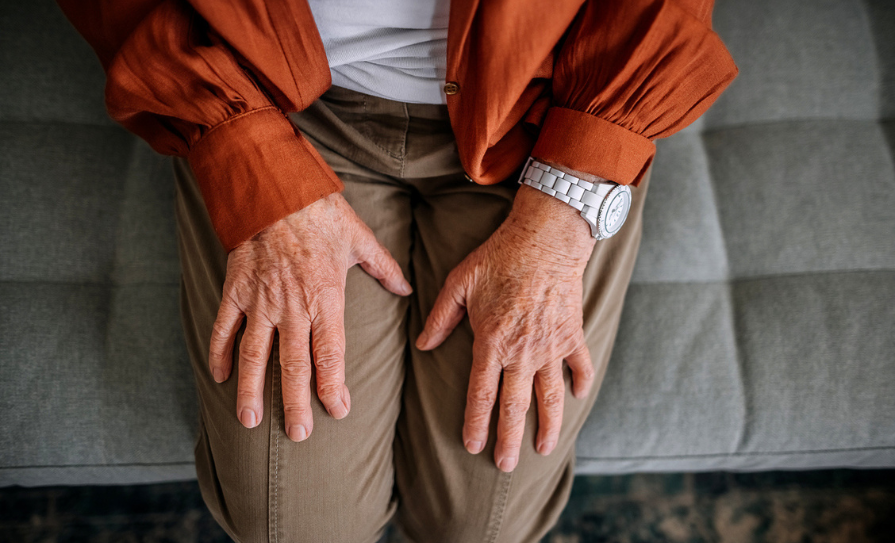